In this issue, I’m digging deep into the analysis layer to answer a question that keeps coming up again and again:
Can a Business Intelligence (BI) tool be used in conjunction with a Product Analytics (PA) tool?
The analysis layer of a modern data stack comprises both Product Analytics and Business Intelligence as neither is a substitute for the other — they both serve different purposes and cater to different teams.
Therefore, the answer to the question of whether the two can be used together is a resounding yes!
Let’s individually look at the two, the teams that benefit from each, and how they can be used together.
Product Analytics
The core purpose of a product analytics tool is to analyze user behavior and understand how users interact with your product.
The data powering Product Analytics tools comes in the form of event data from your website and apps. You can also send event data from external apps but doing so bloats PA tools with too many events and can also result in data quality issues.
Product Analytics tools primarily cater to Product and Growth teams where:
- Product teams are able to improve product development by prioritizing features that are used and killing the ones that are not
- And Growth teams are able to improve onboarding, activation, and retention by visualizing the user journey and identifying points of friction
Mixpanel, Amplitude, and Heap are leaders among Product Analytics tools whereas PostHog (open source) and Indicative (acquired by mParticle) are also popular. They all serve the same purpose and all of them integrate with popular CDI solutions (like Segment and RudderStack).
PostHog is an open-source alternative to Heap as they both offer codeless event tracking, also known as implicit tracking or autocapture.
Indicative is warehouse-centric with support for popular data warehouses (like Snowflake, Google BigQuery, and AWS Redshift) as well as Snowplow as data sources.
With the rapid adoption of the data warehouse, more and more companies are experiencing the benefits of making the warehouse the source of data for both PA and BI tools (more on this below).
Business Intelligence (BI)
When data teams talk about analysis, they generally refer to the analysis carried out in a BI tool that sits on top of a data warehouse. While Data Analysts are the ones who manage BI tools and build reports using the data stored in a warehouse, anyone who can write SQL can essentially use a BI tool.
Unlike Product Analytics tools that rely on event data, BI tools can be used to analyze all types of data, thereby catering to pretty much every team in an organization (including Product and Growth).
While too many to name, the most popular BI tools include Looker, Mode, Tableau, Power BI, and SiSense. And open-source alternatives worth mentioning are Metabase, SuperSet, and Redash.
It’s worth noting that a lot of companies substitute a BI tool with a visualization tool like Google Data Studio for reporting purposes, but doing so takes away the flexibility from SQL-literate end users to query the data directly to run ad hoc analyses, and one has to put in a request for every new report they need which often takes weeks for overworked data analysts to fulfill.
Product Analytics and BI: the estranged siblings?
It should be evident that for data-forward companies, there is a strong need for both types of analysis tools — Product Analytics and Business Intelligence, and one cannot serve the purpose of the other.
Some people have asked why a BI tool cannot do what a Product Analytics tool does and the answer lies in the fact that the data model of standard BI tools is not meant for event data analysis — even a simple funnel analysis (that takes minutes in a PA tool) is quite complicated and time-consuming to achieve in a BI tool.
On the other hand, implementing a Product Analytics tool has always relied on a separate data pipeline where event data is sent directly to the Product Analytics tool via their SDK or an external tracking tool (or a CDP). In fact, most data teams consider Product Analytics a pain as it doesn’t naturally fit into their workflow of collection → warehousing → analysis.
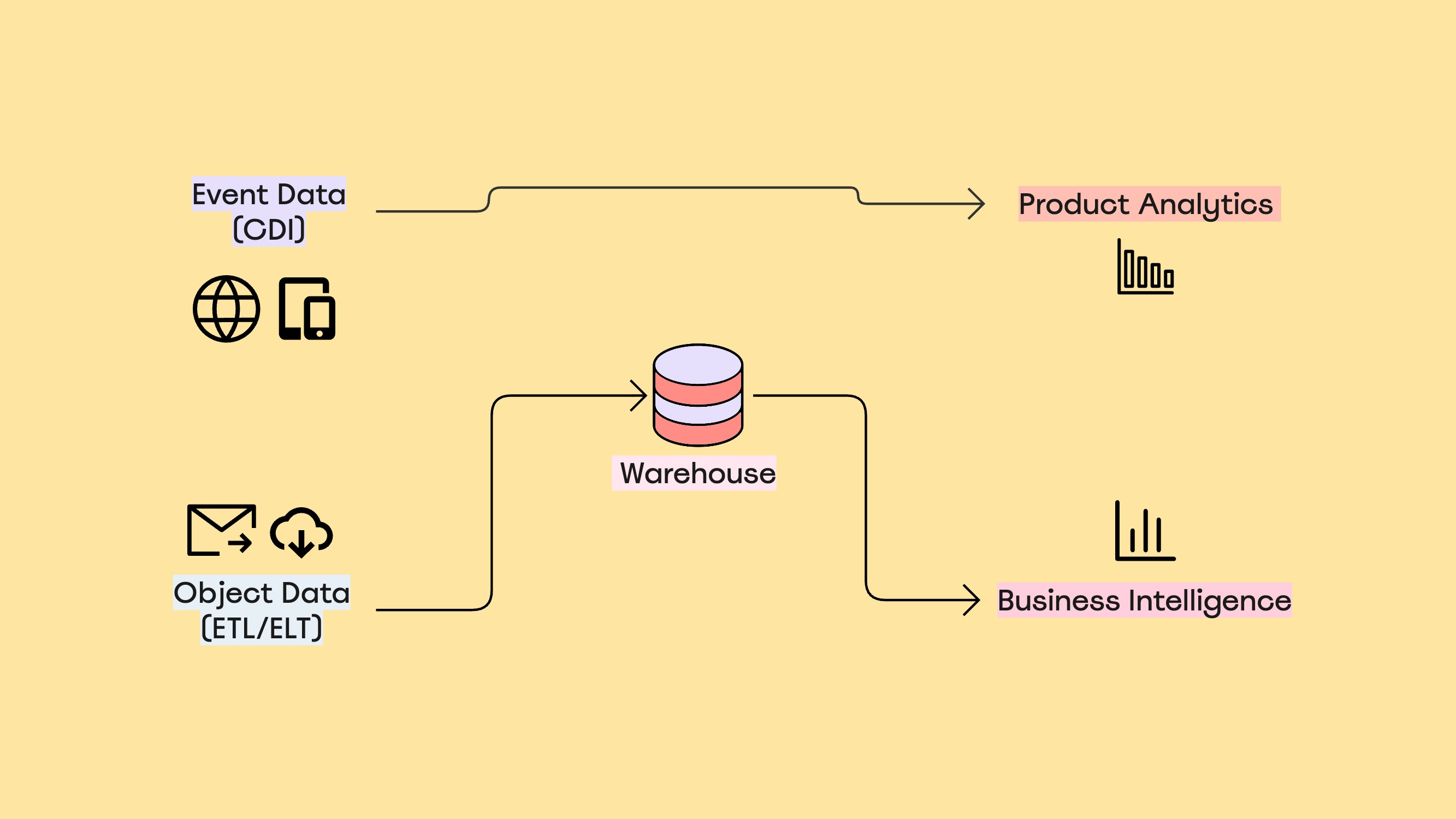
However, under the new warehouse-centric approach where the data warehouse is the data source for both Product Analytics and BI tools, data teams are finally embracing Product Analytics.
And this is also fueling the rise of Reverse ETL tools (like Census and Hightouch) that enable you to sync data from a warehouse to external tools including Mixpanel and Amplitude.

Finally, with reduced data inconsistency between PA and BI, it has become a lot easier for teams to use them together as they don’t have to waste hours figuring out why the same data is telling two different stories!
2022 updates:
- All major Product Analytics vendors now support the cloud data warehouse as a data source.
- A new breed of warehouse-native product analytics tools like NetSpring and Kubit has emerged to fundamentally reimagine the analysis layer of the modern data stack.
- The long-lasting conflict between Business Intelligence (BI) and Product Analytics tools is nearing its end.

