Streaming data infrastructure is going mainstream.
Technologies built to cater to the needs of large-scale organizations like LinkedIn and Uber can now be utilized by startups to deliver hyper-personalized product experiences in real time.
However, making the case for streaming data infra and figuring out where to get started is not trivial. Thankfully, one of the best minds in the space, Dunith Dhanushka is here to help by answering some fundamental questions.
P.S. If you'd like to dig deeper into streaming and real-time analytics, Dunith has curated some great content on the DB forum and is happy to answer follow-up questions too.
P.P.S. The folks behind Startree built the open source real-time OLAP data store, Apache Pinot at LinkedIn. Every time you click on the "who's viewed your profile" button on LinkedIn, it is Pinot that runs a complex query to present that data to you in real time.
Let’s dive in:
Q. In simple terms, what is streaming data infrastructure?
First, I’d like to introduce you to events. Events come before streaming and represent facts about what has happened in the past. When we sequence these events into a stream, we call it streaming data — the infrastructure needed to capture, process, and make sense of events in real time.
Q. What are the prerequisites in terms of the data stack to set up streaming or real-time data pipelines?
Once you look at streaming architecture or the landscape from a high level, you can identify so many components. So we can categorize them based on their role and their responsibility.
- The first step is to produce events from your existing applications or operational systems which is done via SDKs or middleware tools.
- Secondly, you need a scalable medium to store these events and this is where real-time streaming platforms like Kafka and Pulsar come in.
- The third step is what we call “massaging” the data.
We have event producers and then the events are ingested into an event streaming platform, post which the events landing there need to go through some transformation because this is raw data we’re talking about.
It could contain some unwanted information and you often need to mask PII data, or sometimes map JSON into XML, or join two streams together and produce an enriched view, etc. This is what we mean by data massaging.
- In the fourth step, you need a serving layer to present or serve this aggregated or processed information to your end users — internal customers like analysts or decision makers inside your organization, or external users of your product.
In the case of external users, you need a real-time OLAP database or a read-optimized store to deliver real-time experiences.
Those are the four critical components that you need to set up your streaming infrastructure, but this could certainly vary based on the complexity of your use cases.
Q. So what exactly is Apache Pinot and what does it do?
Apache Pinot is a real-time OLAP database.
There are two things to note here — Real-time and OLAP (Online Analytical Processing).
Real-time indicates that Pinot can ingest data from streaming data sources like Apache Kafka, Kinesis, and Pulsar, and make that data queryable within a few seconds.
More importantly, Pinot makes it very fast to run complex aggregated OLAP queries, like the queries that scan multiple batches of data and run complex aggregations with sub-second latency and consistency, tuned for user-facing analytics.
Q. Can you give us a common example of user-facing analytics?
Yes. On LinkedIn, you'll get a notification, in real-time each time someone views your profile, right? That feature is called, "Who viewed your profile" and Pinot is what powers it.
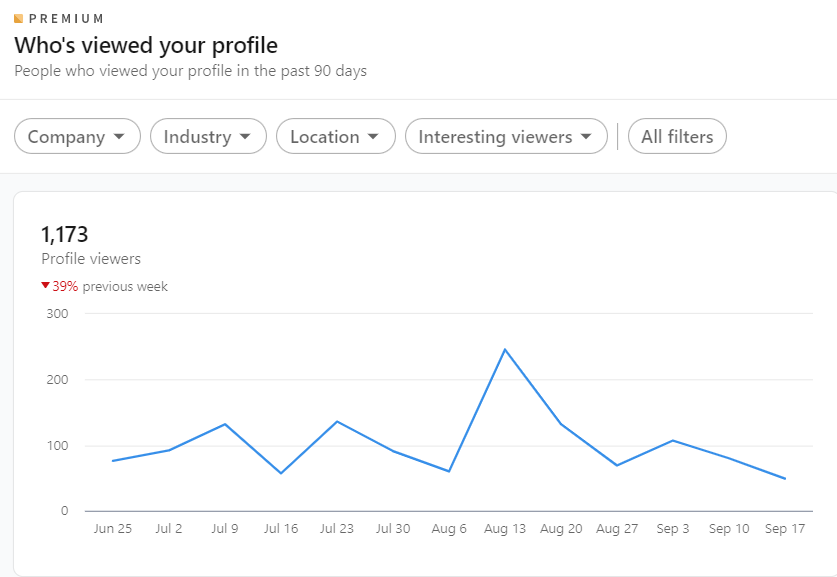
That feature might seem simple but there are lots of complicated things going on to make it happen.
Pinot has to ingest real-time click or profile visits from all the front-end processes, store the data in scalable storage, and then run queries in real-time to answer lots of concurrent questions.
If you look at the scale of those queries, there can be multiple hundreds of thousands of queries executing on the database simultaneously.
Q. What are the benefits of a real-time OLAP data store like Pinot over a regular data warehouse?
There are two main factors that differentiate Pinot from a data warehouse — Latency and Freshness of data.
Pinot can consistently produce queries over sub-second latencies, usually milliseconds. On the other hand, since data warehouses are tuned for internal use cases like exploratory analysis and BI, they produce single-digit latencies most of the time — seconds basically.
Secondly, with Pinot, the freshness of data can be maintained by ingesting from data sources in real-time, whereas with warehouses, one has to employ scripts or ELT tools to batch and load data into the warehouse periodically (on a schedule).
Q. Typically how big or small are data teams at companies that successfully implement streaming or real-time data infrastructure?
It actually depends on the complexity and the velocity of your data infrastructure — how fast you want to process your data and how complex your ecosystem is.
Let's say, you want to build a real-time dashboard for some product for which you can start with one data engineer assuming that all the ecosystem components are available as managed cloud services.
Then as your data velocity grows and your requirements grow, your product grows as well — you can then horizontally scale your team where some people can work on the serving layer, some can work on the stream processing, and others can work on data ingestion, and so on.
Q. How do data adjacent teams like Product and Growth, utilize steaming data that is usually stored in something like Pinot?
We see many use cases related to growth analytics and product metrics — especially at SaaS companies and product-led companies that use Pinot to capture and store their product and engagement metrics.
For example, the first step is to instrument the product using an SDK to emit data points as events into a certain streaming data platform, post which, we can configure Pinot to ingest from that data platform.
Once we have a fresh set of user engagement metrics such as button clicks and page views, we can utilize this vast set of data to understand user behavior.
Product teams can run analyses to derive metrics like DAUs (daily active users), or perform funnel analysis to understand points of friction and to calculate conversion rates. They can plug this data into a BI tool to build real-time reports while Pinot ensures that data is fresh and relevant.
Pinot can essentially help run these analyses really fast, while the data is still fresh and relevant — that’s what Pinot really excels at.
Q. Last question — what’s your one piece of advice for companies just getting started on their real-time data journey?
Real-time analytics is about processing data as soon as it's available.
Which means you need to put many things into consideration. For example, if you’re processing data pipelines with millions of events coming in per second, you need scalable and reliable computing and storage platforms — or infrastructure to process and make sense of those events.
You need to keep in mind that you’re dealing with complicated machinery when it comes to real-time analytics.
Real-time technologies today are getting very cheap with several managed services that make it viable to implement a streaming infrastructure without a lot of resources or technical know-how for simpler use cases too.
But you must first identify your real-time use cases.
If all you need is to populate a dashboard on a daily basis, you can easily get that done with either a data lake or a warehouse with a simple ETL job.
But then again, there can be some complex use cases such as anomaly detection, real-time recommendations, and real-time dashboards that require careful planning in terms of storage, computing, and analytics infrastructure.
To summarize, know your use case better and think of how much complexity and budget you can spend on that use case.
🥁🥁
You can also tune in to the episode on Spotify or Apple Podcasts.
Ready to keep learning about real-time analytics? Next up — Change Data Capture, a critical component of streaming data infrastructure.

