🥁 This episode is brought to you by NetSpring, a Warehouse-native Product Analytics tool. 🥁
🥁🥁
The future looks bright — one where the long-lasting conflict between Business Intelligence (BI) and Product Analytics is finally resolved!
For data-forward companies, there is a strong need for both types of analysis tools as they were built to work with fundamentally different types of data — BI for relational data and Product Analytics for event data — and serve fundamentally different purposes.
BI is an analysis interface for the entire organization, enabling stakeholders to derive insights from the analyses performed by data teams.
Product Analytics, on the other hand, has proven to be non-negotiable for teams to better understand product usage and identify points of friction in the user journey.
In reality though, the user journey extends well beyond the core product (web and mobile apps) — it includes interactions a user has with a brand across engagement, advertising, and support channels.
Teams need to combine product-usage data with data from third-party tools to get a complete picture of the user journey. But doing so has been rather challenging using first-generation Product Analytics tools, and even more so using BI tools.
Enter Warehouse-native Product Analytics.
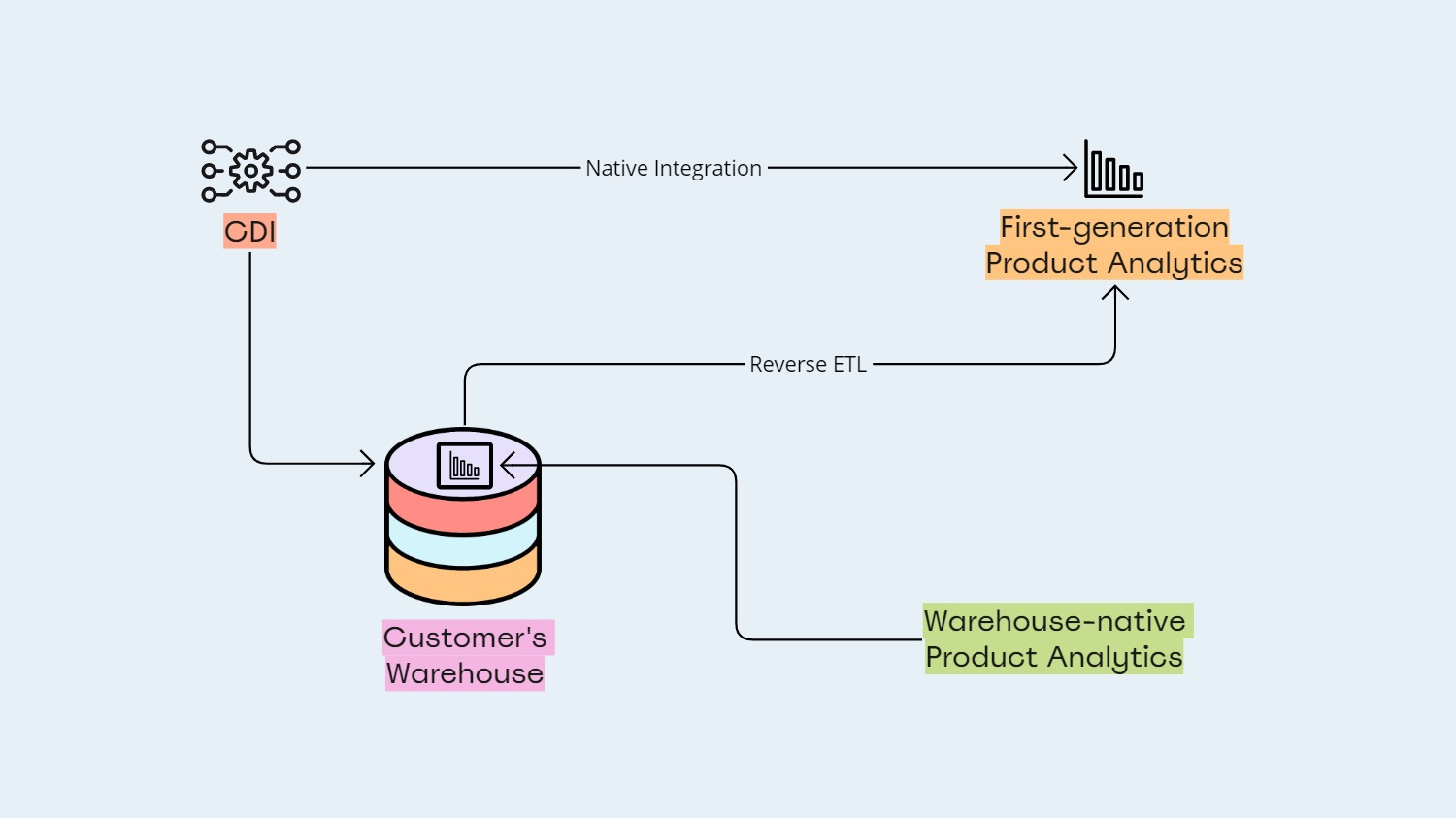
A Warehouse-native Product Analytics tool sits on top of the customer's data warehouse, allowing teams to perform end-to-end analyses that combine first-party behavioral data with data from third-party sources used for email engagement, advertising, and support.
In this episode, Vijay Ganesan, CEO of NetSpring walks us through the ins and outs of this technology (Warehouse-native Product Analytics) via answers to questions like:
- Why has it been challenging to offer both BI and Product Analytics capabilities in a single product? How is that changing with the rise of the cloud data warehouse?
- What are the organizational shifts contributing to the adoption of warehouse-native apps?
- How does the warehouse-native approach better equip organizations to comply with privacy regulations like the GDPR?
If you work in Product, Growth, or Data, I’m certain that you’ll find this conversation insightful.
Watch the full episode on LinkedIn
You can also tune in on Apple, Spotify, Google, and YouTube, or read the key takeaways from the conversation below (slightly edited for clarity).
🥁🥁
Key takeaways from this conversation
Arpit (02:06):
BI was built to explore relational data, whereas Product Analytics relies on event data or behavioral data. From a technical point of view, why is it so challenging to offer both BI and Product Analytics capabilities in a single product?
Vijay (02:20):
Historically, the two worlds have been very distinct at all levels of the stack.
- The way you collect and store data is very different for BI and Product Analytics: High-velocity event data typically never reached the data warehouse — it wasn’t feasible to store petabyte-scale data in traditional data warehouses anyway.
- The nature of computation is very different: In BI, you're doing dimensional slice and dice — you take a metric and slice it by different dimensions. The way you structure the data for BI is very different from what you do for Product Analytics where you’re not studying the final state — you're studying the sequence of events that lead to a final state.
- The way you express the analytical computations are very different too: In BI, it's SQL oriented whereas, in the event data world of Product Analytics, SQL is not the best language to express the analytical intent.
Therefore, at all levels, BI and Product Analytics are very different types of systems, making it very difficult to do one in the other.
Arpit (06:50):
NetSpring doesn't ingest any data but are there any prerequisites for it to work? Do companies need to model their event data as per a specific schema?
Vijay (07:06):
We don't do any instrumentation as we believe in the concept of decoupled instrumentation — the idea that you use best-of-breed, unopinionated instrumentation systems (CDIs) like Rudderstack, Segment, or Snowplow to land the data in the data warehouse in a form that is consumable by anyone.
Secondly, in terms of schemas and data models, and this is one of our key differentiations, we can consume any arbitrary schema. Unlike first-generation tools (like Mixpanel and Amplitude), we don't require you to force your data model into some pre-canned user event — we can work off a generic data model.
And this goes back to our BI DNA where we're similar to BI tools in the sense that they can work off arbitrary schemas.
We're fundamentally relational in nature with event layered on top of it which we refer to as Relational Event Streams — you point NetSpring to whatever schema you have in your data warehouse, do some decorations on certain data sets to turn them into event streams, and then you have the full glory of product analytics and sophisticated BI-type analytics on top of the data warehouse.
Arpit (08:31):
Today, all first-gen product analytics tools support the data warehouse as a data source, but they still need to store the data in their own environment. Besides the lack of ingestion, is there anything else a warehouse-native product like NetSpring does differently?
Vijay (09:36):
When we talk about working off the data model in your data warehouse, we talk about consuming those data models in their native form. On NetSpring, if you're looking at Salesforce data, for example, you'll see first-class entities for accounts, contracts, and opportunities. Similarly, with Zendesk, it's tickets. The ability to consume this business context in a native form is very powerful, and that's what lends itself to very rich analytics.
Arpit (10:42):
And what are the key factors that make warehouse native products more affordable than their traditional counterparts?
Vijay (11:38):
Besides the fact that you don’t pay twice for your data with a copy in the warehouse and a copy elsewhere,
- You need not pay for Reverse ETL jobs to move data from the warehouse to the Product Analytics tool
- And there’s a cost associated even with figuring out what to send, what to not to send, what to delete, etc — you don't have to worry about any of that.
So there's process cost, operational cost, and then there is a large opportunity cost. If your analytics is siloed and not impactful, the opportunity cost is huge.
With warehouse-native tools, you only pay when someone queries the data.
Therefore, the overall difference in cost between the first-generation approach to Product Analytics and the warehouse-centric approach is significant — just like when you went from Teradata to Snowflake, there was an order of magnitude difference in cost. It's the same thing. It's a generational shift.
Arpit (12:37):
With product analytics running on the data warehouse, it becomes really easy for go-to-market (GTM) teams to access all types of data and perform richer analysis.
For instance, I can now combine product usage data with data from my email engagement tool to get a full picture of the user journey — how users are moving from doing something inside the product to actually engaging with an email campaign, and then performing the desired action by going back into the product, or not.
As someone who ran growth, I cannot stress on how important it is for teams to perform such analyses.
Vijay, what else would you add here?
Vijay (13:21):
We talk about this concept of evolving from product analytics to business analytics. Historically, product analytics has been siloed. But, the world is changing towards the product becoming the center of the business — the primary driver for growth.
Therefore, we believe that companies need to move from product metrics to business metrics, where product instrumentation data needs to be combined with data from other business systems to get an end-to-end view of the customer experience.
Arpit (14:06):
Besides the rise of the data warehouse, what other organizational shifts are contributing to the adoption of warehouse-native apps?
Vijay (14:14):
A combination of three key trends is driving a generational shift in product analytics:
- Warehouse-centricity where all the data comes into the warehouse
- Composable CDPs
- Increasing sensitivity toward security and privacy where the tolerance for data going off into some black hole is going away very fast.
Arpit (14:59):
Lastly, how does the warehouse-native approach to product analytics make it easier for organizations to adhere to privacy regulations such as the GDPR?
Vijay (15:06):
It's very simple.
Being warehouse-native fundamentally implies that no data leaves your secure enterprise environment. It's almost a religion for us that data will never leave.
Building caches, materializations, indexes, and cubing systems outside of the data warehouse to increase performance — we don't do any of that stuff. We believe you can push everything (all data) into the warehouse and still get very good performance.
Ultimately, if the systems that plug into the warehouse don’t make copies of the data, it’s much easier for organizations to be in compliance with privacy regulations.
🥁🥁
Have questions for us or insights you’d like to discuss? We’d love to hear from you on LinkedIn.
Ask questions or share your thoughts
Additional Reading

