This is part 5 of a 5-part series titled Data-powered Messaging For True Personalization – powered by Piwik PRO.
Today, people’s needs and preferences are changing at a rapid pace, especially in the face of new information becoming available so quickly. What I liked yesterday I might despise today, and vice versa. Browsing a product or buying it once is a poor indication of me wanting more of that product. Clicking on a notification or opening an email doesn’t necessarily indicate interest – I might do it out of curiosity, boredom, or even by mistake.
Therefore, it’s not always enough to personalize messaging based on a user’s past actions and transactions – or first-party data.
So what else is needed?
Preference data, also known as zero-party data, collected continuously from users to capture their changing preferences.
Both first-party and zero-party data help deliver personalized messages; you may refer to this guide that covers the differences between the two.
Now, before going into some use cases depicting the benefits of preference data, I’d like to go over the three main consequences of ignoring it.
The consequences of ignoring preference data
By relying solely on first-party data (collected implicitly) and not complementing it with zero-party data (shared by users explicitly), brands let go of the opportunity to build better relationships with their diverse audiences.
More specifically, here’s what happens when brands ignore zero-party data:
- Personalization is presumptive
- Ad dollars are wasted
- Privacy and compliance go for a toss
Personalization is presumptive
When brands rely too heavily on first-party data, all their data-powered campaigns are based on past transactions and actions – behavioral data that’s usually days or weeks old by the time it’s acted upon.
In other words, behavioral data represents what a user has done in the past, which alone is rarely a reliable indicator of what they need or want in the present. Therefore, personalization that solely depends on behavioral data is presumptive in nature as it doesn’t account for user preferences.
On the other hand, a combination of behavioral data and preference data gives a more accurate picture of a user’s needs since the latter is shared by users directly. Compared to behavioral data alone, this combined data set is more dependable and will likely lead to better messaging campaigns.
It’s also worth mentioning that behavioral data can be inconsistent or incomplete due to outages or tracking bugs; the process of collecting behavioral data is non-trivial and a lot can go wrong if the implementation is not well-planned. (If you’re looking to get started with behavioral data collection, you’ll find this guide helpful).
Ad dollars are wasted
It’s mind-boggling how much money brands waste by showing irrelevant ads to people, isn’t it?
Just because someone visited my website or viewed one of my products, following them around the internet will result in a sale – a tactic that might have worked in the past but in the age of AI and hyper-personalization, this seems like an easy but lazy thing to do.
I say this because most brands are not putting enough effort into understanding what users want – by explicitly asking them questions like:
- What are you looking for? Do you like what you see? Did you find what you’re looking for?
- Are you interested in this product? Are you buying for yourself or someone else? Would you like us to recommend similar products?
- What’s your favorite color? What’s your favorite apparel brand? And your favorite footwear brand?
Amongst the brands collecting such valuable zero-party data, most fail to utilize it to inform their ad campaigns. Heck, most brands don’t even stop showing ads to converted users, which is a clear sign of poor personalization efforts.
But what if that’s not the case? What happens when a brand successfully uses preference data to improve its advertising campaigns? Well, the ads ought to be more relevant and will likely lead to more conversions.
For instance, equipped with rich preference data, the marketing team can show me ads about the products I actually like or brands that I already love. Doing so is as easy as creating segments and syncing them to Google Ads or any other ad platform. As an added benefit, the data can also be used to reach more people like me with similar preferences via lookalike audiences.

Also, once I go ahead and make a purchase, I can easily be excluded from the corresponding campaigns by syncing purchase data to the ad platform. This also informs the brand which of their ads in a campaign are working, enabling them to optimize the copy and creative for other campaigns too.

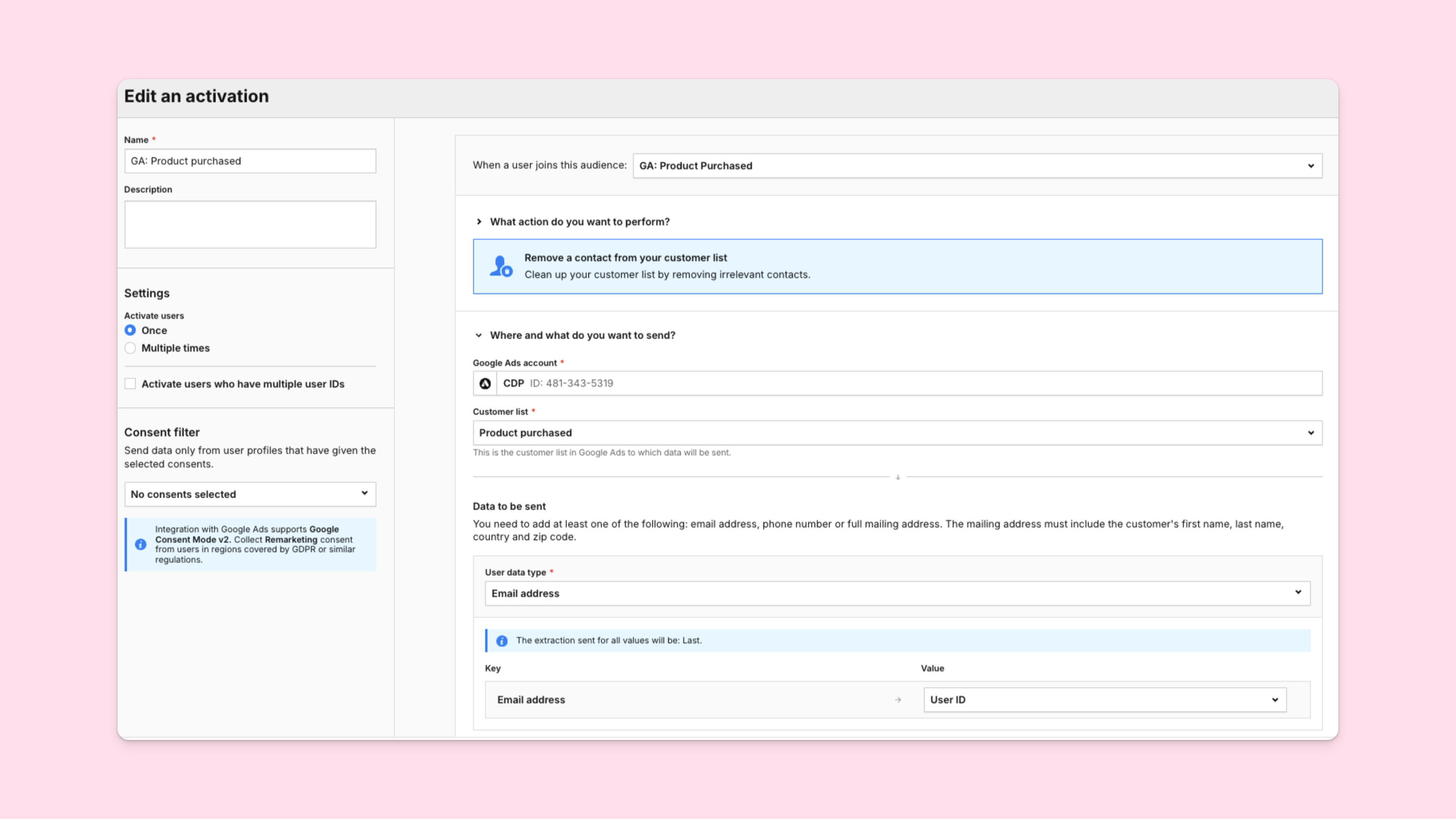
Collecting preference data and syncing them accurately to ad platforms is certainly a good amount of work, but at the same time, this data can be a goldmine for better advertising, moreso as third-party cookies are phased out and brands have to rely on first-party and zero-party data.
Privacy and compliance go for a toss
Every brand aspires to deliver personalized messages and content throughout the customer lifecycle from acquisition to retention and beyond. However, delivering communication based on assumptions derived from a user’s actions is the definitive way to violate privacy regulations.
Here are some examples:
- If someone joins our community, we must invite them to all our future events.
- If someone registers for an event, we must send them emails asking them to try our product.
- If someone signs up for a trial, we must send them case studies, product updates, company updates, and of course, event invites.
Besides a colossal waste of resources, the above approach prevents brands from finding out who their audiences really are, why they joined the community or registered for that event in the first place, and what kind of content they wish to consume.
Use cases depicting the benefits of preference data
It’s time to look at some use cases depicting the benefits of including preference data in messaging campaigns.
Self-consumption vs. gifting
When a user is buying, say, women’s jewelry, it’s useful to find out whether they’re buying for themselves or their partners by capturing the user’s gender and intent data in the purchasing flow.

Doing so enables the brand to deliver cart abandonment messages that are truly personalized. Also, it makes it easier not to deliver irrelevant messages in the future – which is also a form of personalization.
Preferences vs. behavior
A user’s browsing behavior and their specified preferences might not tell the same story, indicating either a change in one’s preferences or shared accounts. In such cases, preference data should be prioritized over behavioral data since it reflects the primary user’s current needs.
Celebratory offers
Not everyone appreciates celebratory messages and offers that brands deliver on occasions like Mother’s Day, Father’s Day, Christmas, and so on. Brands that truly care about empathetic engagement and wish to build stronger relationships with customers must enable users to easily turn on or off celebratory messages – ideally from a preference center.
There’s so much more brands can do if they invest in the continuous collection of preference data, just like they invest in the continuous collection of behavioral data.
Now let’s take a quick look at the various tools brands can use to collect preference data.
Tools to collect preference data
Preference data, as mentioned earlier, is also known as zero-party data and includes any piece of information that a user shares intentionally or explicitly with a brand.
When someone signs up for a product, they typically share some personally identifiable information (PII) such as their full name, email address, and phone number. If they’re buying a physical product, they share their mailing address at the time of checkout.
B2C services usually ask for demographic information like age, gender, and location whereas most B2B vendors seek professional information including the size of the company the user represents, the industry they belong to, and their role, function, or job title.
Some B2B and many B2C brands, especially the ones that are serious about personalization, collect preference data by asking users for more specific information such as:
- Their needs or the problems they’re trying to solve
- The products they’re interested in
- The types of messages they’d like to receive
- Their favorite brand, vacation spot, and so on
These data points, collectively referred to as preference data or zero-party data, are collected via the following tools:
- Surveys
- Preference centers
- In-app widgets
- Communication tools

The biggest challenge here is that preference data is usually unstructured since it’s collected using a variety of methods and tools, with little to no standardization or predefined taxonomies. Therefore, the key is to ensure that the data is collected and stored in a structured manner, ready to be used for segmentation purposes.
Once you have all this data available, use it to build segments and sync them to your engagement and advertising tools to deliver truly personalized experiences.
Try this today
Conduct the following exercise to put what you’ve learned into action:
- Conduct an audit of the various touchpoints in your product where there’s a potential to collect preference data
- List the top two use cases for those data points and then proceed to list the questions you’d like to ask users at the respective touchpoints
The possibilities are endless but at the very least, you must try to collect preference data during these: onboarding, upgrade, downgrade, subscription to any type of communication (notifications, emails, etc).
Good luck!

